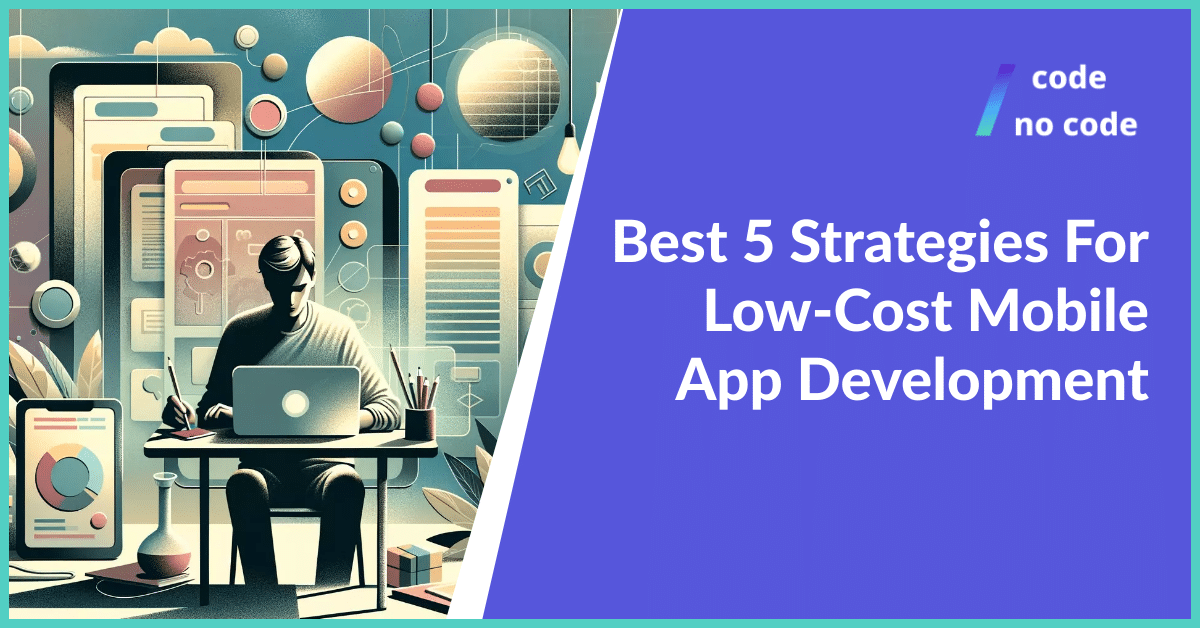
Best 5 Strategies For Low Cost Mobile App Development
Mobile app development and app development in general is not cheap. There are a lot of factors to consider and it can be just a headache to...
In the dynamic landscape of modern business, the integration of Artificial Intelligence in customer service has marked a revolutionary shift. This evolution has given rise to the concept of “machine customers,” a term that encapsulates the automated, AI-driven systems and interfaces that interact with human customers.
The emerging phase of machine customers, or custobots, reflects an era where technology not only supports but also actively participates in consumer and business purchases. This trend, bound to bloom in 2024, according to Gartner, is fueled by the growing need for efficiency, personalized experiences, and data-driven decision-making in customer service.
As AI software development continues to advance, custobots are becoming increasingly sophisticated and capable of understanding and responding to a wide array of customer needs and behaviors. This progression to autonomous customers is not just a technical leap. It is reshaping the very fabric of how businesses interact with human customers, making understanding machine customers an essential aspect of any forward-thinking customer service strategy.
The concept of machine customers represents a groundbreaking shift in the realm of customer service and business interactions. In other words, machine customer is a non-human economic actor. Essentially, it refers to an automated system, typically powered by AI, that can make purchase decisions and conduct transactions autonomously, without direct human intervention.
Central to the functioning of machine customers is ML, a subset of AI that enables these systems to learn from data, identify patterns, and make decisions with minimal intervention or involvement of human customers.
This technology allows machine customers to offer personalized, efficient, and informed responses, making interactions more engaging and effective.
Machine customers are transforming customer service by both efficiently completing tasks, providing round-the-clock support, handling large volumes of inquiries simultaneously, and delivering consistent, unbiased responses.
They can be adaptable and improve over time, learning from each interaction to refine their responses and act independently in decision-making processes.
This not only enhances client satisfaction but also frees human staff to tackle more complex, nuanced issues, optimizing the overall efficiency and effectiveness of customer service operations.
In summary, machine customers represent the synergy of AI and customer service, revolutionizing how businesses interact with their clientele by offering smarter, faster, and more personalized support.
In Gartner’s framework, the evolution of machine customers is divided into three phases. At the moment, we are still in the first one. We’re bound to reach the second by 2026 and the third by 2036.
1. Bound Customer: In this phase, machine customers operate within strict, predefined rules and guidelines. Their interactions and decisions are limited to specific scenarios, offering basic, rule-based responses to customer inquiries.
2. Adaptable Customer: Here, machine customers exhibit more flexibility and learning capabilities. They adapt based on customer interactions, using data to improve and refine their responses. This phase allows for more personalized and context-aware customer service.
3. Autonomous Customer: In the most advanced phase, machine customers are fully autonomous, capable of complex decision-making without human intervention. They leverage advanced AI and machine learning to understand, predict, and independently respond to a wide array of customer needs, offering highly sophisticated and intuitive customer experiences.
The impact on AI to customer satisfaction is profound and multifaceted, significantly enhancing interactions between businesses and consumers. AI technologies play a crucial role in understanding and improving client experiences, chiefly through advanced data analysis and automation.
By analyzing customer data, AI systems identify patterns and preferences, enabling businesses to tailor their offerings and interactions for maximum relevance and impact.
One prominent example of AI in action is the use of chatbots. These AI-driven assistants provide instant, 24/7 customer support, handling inquiries and resolving issues with unprecedented efficiency.
Predictive analytics is another powerful AI tool, that forecasts customer behaviors and preferences, thus allowing businesses to proactively meet customer needs.
Additionally, AI facilitates personalized recommendations, offering customers tailored suggestions based on their past interactions and preferences, thereby enhancing overall client satisfaction.
These AI applications not only streamline operations but also foster deeper, more satisfying customer engagement.
Machine customers are already widely used. Gartner predicts that by 2028, 15 billion connected products will exist with the potential to behave as customers, with billions more to follow in the years ahead. Here’s a list of real-life examples where custobots are already autonomous customers:
These examples demonstrate how machine customers are not just theoretical concepts but are actively being used by major brands to drive innovation and efficiency in their operations. The use of AI, IoT, and automation in these scenarios represents a significant shift in how businesses operate and interact with their supply chains, clients, and markets.
The incorporation of machine customers in business has ushered in a new era of efficiency and accuracy, particularly in client service and data analysis. By leveraging AI-driven systems, businesses experience a significant enhancement in operational efficiency.
These machine customers automate routine tasks, such as handling customer inquiries and managing service requests, which reduces response times and elevates client satisfaction.
This automation not only streamlines customer service processes but also allows human employees to focus on more complex and creative tasks, thereby optimizing workforce utilization.
Additionally, machine customers excel at analyzing vast amounts of customer data with unparalleled accuracy. They employ advanced algorithms to sift through data, identifying patterns and insights that might elude human analysis.
This leads to more informed and precise decision-making. By accurately predicting customer behavior and preferences, businesses can tailor their strategies and offerings more effectively, resulting in improved client engagement and business outcomes.
The synergy of machine customers in these domains fundamentally transforms how businesses interact with their clientele, driving both innovation and growth.
ML, a critical component of AI, plays a pivotal role in enhancing customer interaction and analysis. By utilizing ML algorithms, businesses gain deep insights into customer behavior, preferences, and needs. These algorithms analyze vast datasets, including transaction histories, browsing patterns, and customer feedback, to uncover trends and patterns that inform customer-centric strategies.
One prominent application of ML in customer interaction is personalized recommendation systems. For example, online retail giants like Amazon use ML to analyze past purchases and browsing behavior, offering tailored product recommendations that significantly boost customer engagement and sales.
Another example is Netflix’s recommendation engine, which employs ML to curate personalized viewing suggestions based on individual user preferences, enhancing user experience and retention.
In customer service, ML-powered chatbots provide efficient and personalized support, handling routine queries and escalating complex issues to human agents. This not only improves response times but also ensures a more personalized customer service experience.
These successful implementations of ML in customer interaction and analysis demonstrate its capability to transform data into actionable insights, leading to more informed decisions, enhanced customer experiences, increased companies revenue, and ultimately, business growth.
The integration of ML in these domains is a testament to its transformative power in understanding and catering to the evolving needs of customers.
Implementing machine customers in business environments, while beneficial, brings several challenges and considerations. A primary concern is privacy and data security.
As machine customers collect and process vast amounts of customer data, ensuring this information’s security and handling it in compliance with privacy laws like GDPR becomes crucial. Businesses must establish robust security protocols and transparent data practices to maintain customer trust and legal compliance.
Integration complexities also pose significant challenges. Seamlessly integrating AI-driven systems with existing business processes and infrastructure requires substantial technical expertise and resources.
It often involves overcoming compatibility issues and ensuring that these systems can effectively communicate with other business applications, which can be both time-consuming and costly.
Moreover, the efficacy of machine customers hinges on their ability to continuously learn and become adaptable customers. This requires ongoing maintenance, updates, and training of AI models to keep pace with evolving customer behaviors and market trends.
Without this continual adaptation, the effectiveness of machine customers may diminish over time, leading to outdated responses and decreased customer satisfaction.
One more challenge is regulatory compliance. Machine customersmust operate within the legal and regulatory frameworks of their respective industries.
A key consideration for machine customers is reliability and trust. Establishing trust in custobots’ decisions, particularly in critical applications, is essential.
Addressing these challenges is important for businesses to fully leverage the benefits of machine customers while maintaining operational integrity and customer trust.
The future of machine customers is poised for groundbreaking advancements, largely driven by the rapid evolution of AI.
We’re likely to see an increased adoption of AI in customer-facing roles, offering more sophisticated, personalized interactions. Predictive AI will play a pivotal role, enabling businesses to anticipate customer needs and preferences, thereby proactively tailoring services and products.
Another emerging trend is the integration of emotional intelligence into AI systems. This will allow machine customers to understand and respond to human emotions, significantly enhancing customer engagement and experience.
Additionally, the convergence of AI with other technologies like augmented reality (AR) and virtual reality (VR) is expected to create immersive and interactive customer service experiences. This integration could revolutionize retail and e-commerce, offering customers highly engaging and personalized shopping experiences.
Overall, the future of machine customers is marked by more intuitive, empathetic, and interactive AI-driven systems, leading to more effective and meaningful customer interactions.
The integration of AI in customer service, manifesting primarily through machine customers, represents a significant transformation in business-customer interactions. These AI-driven systems, equipped with machine learning algorithms, have redefined efficiency and personalization in customer service.
They offer in-depth insights into customer behavior and market dynamics, leading to more informed and tailored business strategies. The benefits of machine customers in business are manifold, including enhanced efficiency, automated and personalized customer interactions, and improved accuracy in data analysis and decision-making.
However, the implementation of machine customers is not without challenges. Privacy concerns, integration complexities, and the need for continuous learning and adaptation are key considerations businesses must address.
Looking ahead, emerging trends suggest an even deeper integration of AI in customer-facing roles, with emotional intelligence and the combination of AI with technologies like AR and VR poised to further enhance customer experience.
As AI continues to evolve, it’s clear that its role in customer service will only grow, leading to more innovative, efficient, and customer-centric business models. This evolution promises not only improved operational efficiencies but also a more intuitive, engaging customer experience, paving the way for a future where AI is an integral part of every customer interaction.